Tissue morphology influences the timing of cell development in human brain organoids
In this study, we investigated how overall tissue structure influences the decisions cells make about their fate. We took advantage of the variety of methods for making organoids, simplified models of organs grown in the lab, to explore this relationship between form (how the organoid looks) and fate (what happens to the development of cells inside it).
We found that small differences in 3D cell culture protocols affect both the shape and complexity of the resulting organoids, down to details of the tissue architecture. We then examined the impact of organoid morphological changes on cell fate through integrated single nuclei RNA sequencing (snRNA-seq) with the Evercode assay and spatial transcriptomics.
We found that independent of the protocol, organoids with more complex shapes more closely resembled the development of in vivo human fetal brain. In contrast, protocols that disrupted the structure of the organoid, resulted in cells segregating into the wrong tissue or developing along incorrect timelines. Finally, encapsulation to simplify the shape of organoids led to chaotic tissue architecture and abnormal timing of cell maturation.
These results suggest that the spatial arrangement of cells is important for their correct development over time, particularly in the brain.
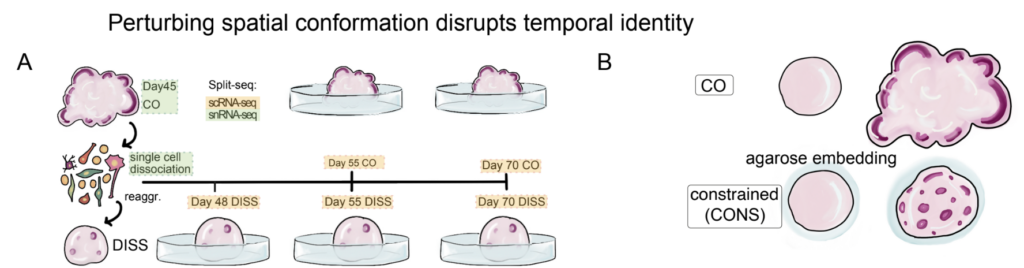
Figure 1. Schematic of Experimental Design. COs at day 45 were dissociated into single-cell suspension and reaggregated, or nuclei were extracted for sequencing. In parallel, single nuclei were extracted from whole CO at day 45 and sequenced. Samples processed for snRNA-seq are highlighted in green. Single-cell suspension was plated to form reaggregates, that were followed over time. Dissociated-reaggregated organoids (DISS) were collected for single-cell RNA-seq at days 48, 55, and 70. In parallel, unperturbed COs were collected for sequencing at days 55 and 70. For each sample there are three batches with two biological replicates per batch, per time point. For day 45 nuclei, day 48 single cells and day 55 single cells, two batches with two biological replicates were collected for sequencing.
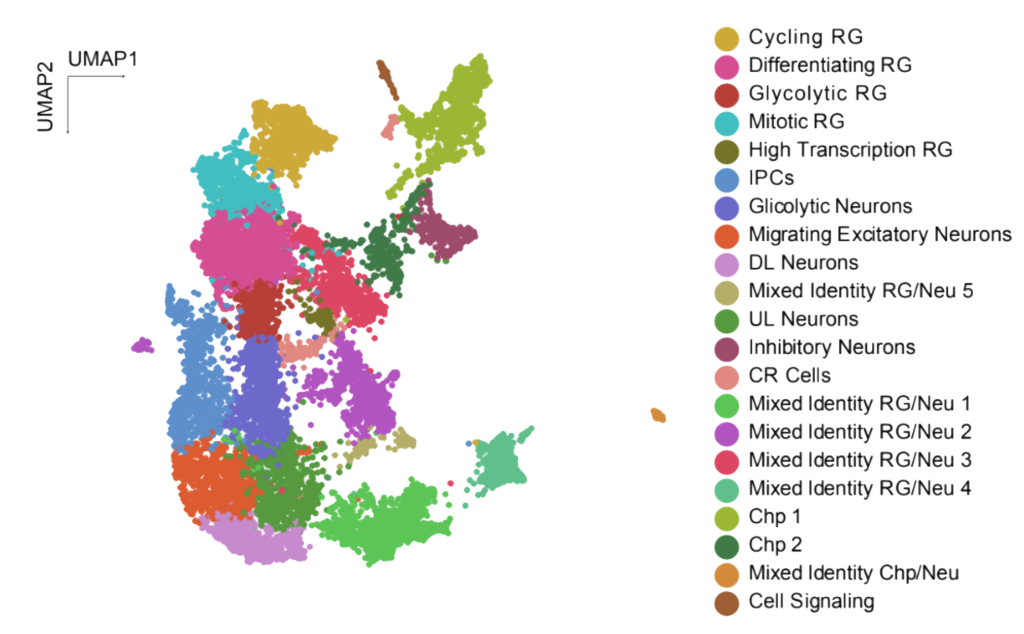
Figure 2. snRNA-seq UMAP of day 55 CO, CO noMG, FAST, and DISS organoids. Cells are colored by clusters. Three batches with one organoid each per condition were sequenced, except for CO noMG condition with three organoids from two batches.
Next Steps
- See publication for details: Chiaradia et al. Tissue morphology influences the temporal program of human brain organoid development. Cell Stem Cell. 2023 Oct 5.
- Explore the data
- Download the R files (below).
- The data was processed according to the Material and Methods described in the referenced paper.
- There are two SingleCellExperiment objects to be read in R. These may be analyzed using the “scran” R package in addition to several other packages for single cell transcriptomic analysis.
- The scRNAseq (cell) data includes counts, decontaminated counts from Xdecont and the corresponding log normalized counts. The PCA and UMAP were used to generate the paper results. Additionally there is extensive cell and gene metadata included.
- The snRNAseq (nuclei) data contains everything as the previous folder but as there is no need to apply a decontamination procedure – raw and normalized counts can be used directly for downstream analysis.
- See the README file included for a description of the data.
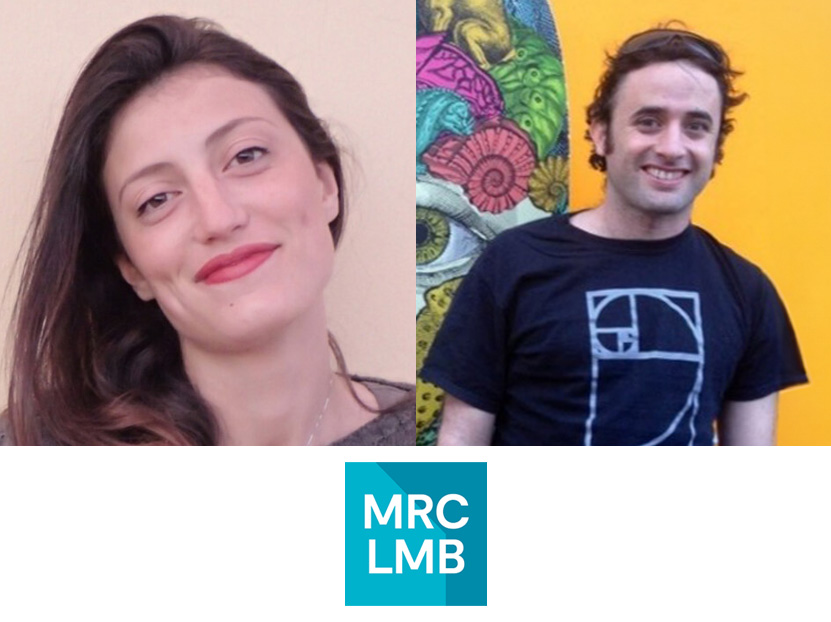
"Evercode is great for time course experiments, the batch effect nightmares disappear."
We're your partners in single cell
Reach out for a quote or for help planning your next experiment.